Chapter 1 Introduction to Single Cell Technology
1.1 Single cell RNA sequencing (scRNA-seq)
Single cell RNA sequencing (scRNA-seq) encompasses a range of technology to generate genome-wide expression of many individual single cells.
Starting from tissue samples we get, we will get the analysis results after following processes.
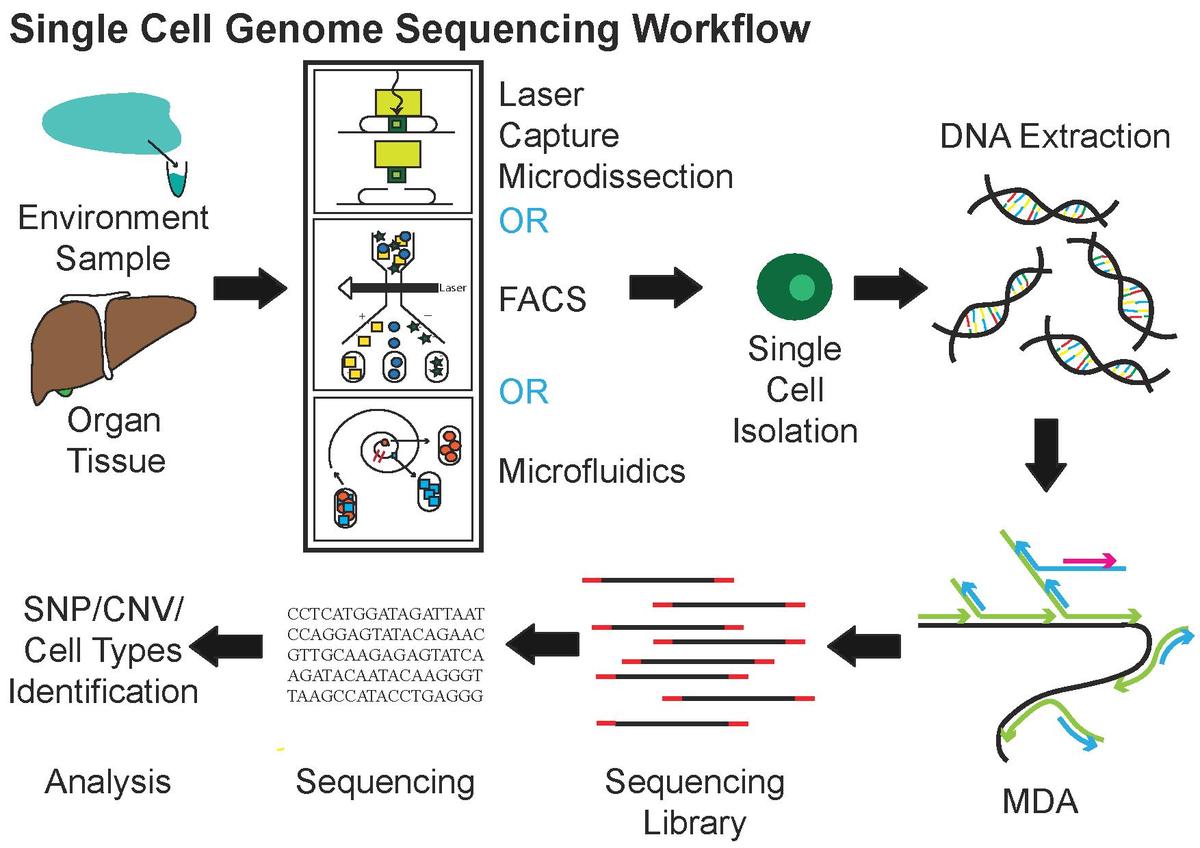
Figure 1.1: Single Cell Genome Sequencing Workflow (taken from Wiki). MDA: multiple displacement amplification
1.2 Cell isolation
Droplet-based:
tissue sample must be dissociated into suspension
cells will be encapsulated into a water-in-oildroplet individually
high-throughput and low cost
related technologies: Drop-seq (Macosko et al. 2015), inDrop (Klein et al. 2015), Chromium 10X (Zheng et al. 2017)
Non droplet-based:
Smart-seq2 (Ramsköld et al. 2012): manual cell picking with micro capillary pipettes
CEL-seq (Hashimshony et al. 2012): individual cells are added to tubes; the first one introducing barcodes and pooling of RNA
MARS-seq (Jaitin et al. 2014) is the first one using FACS to isolate single cells into individual wells, and the optimized version MARS-seq2 (Keren-Shaul et al. 2019) came out with lower cost, improved reproducibility, reduced well-to-well contamination
The following figure shows some common single cell isolaion techniques (Hwang, Lee, and Bang 2018).
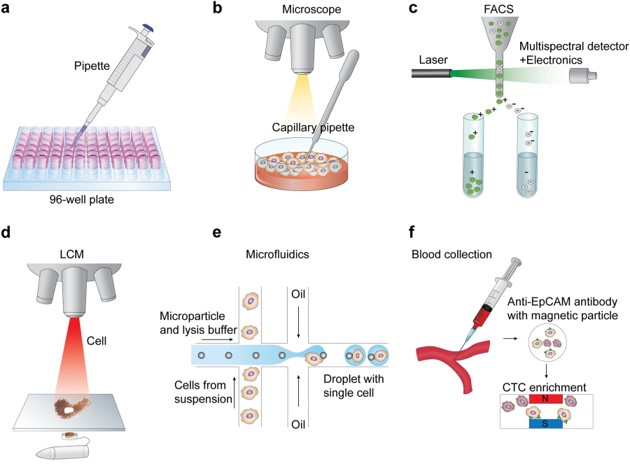
Figure 1.2: Single Cell Isolation (modified from Hwang, Lee, and Bang 2018)
For more information about different cell isolation techniques: Hu et al. (2016)
1.3 Barcodes and Unique molecular identifiers (UMI)1
This part is modified based on the lecture note, Single Cell RNA-Seq - Introduction created by David Tse (2018).
Paired-end sequencing outputs two fastq files corresponding to the 5’ and 3’ direction of sequencing. With this sequencing technology, the first read of the pair always coincides with the cell (barcode + UMI) part of the primer.
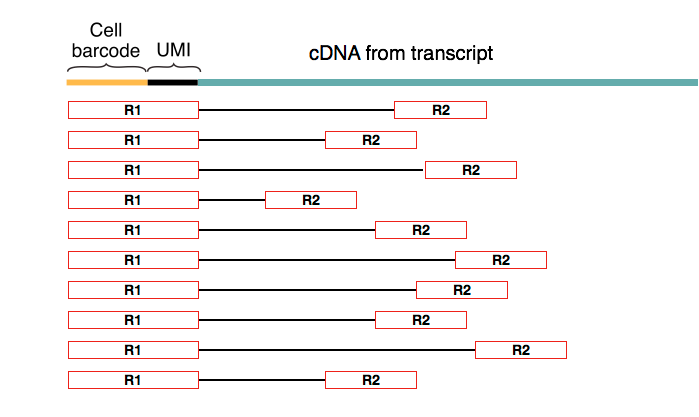
Figure 1.3: Biased paired-end reads (David Tse et.al)
Based on the obtained reads consisting of cell barcode, UMI and cDNA, we can estimate the transcript abundances. This allows the mapping algorithm to distinguish which sequences are barcodes and which are transcript sequences. Thus, it is important to recognize the library preparation chemistry used for sequencing in order to determine cell barcode and UMI barcode sequence length and location.
To get the UMIs’ counts, we can first group reads by cell barcode before aligning cDNA reads and counting unique molecules per cell per gene using the UMIs.
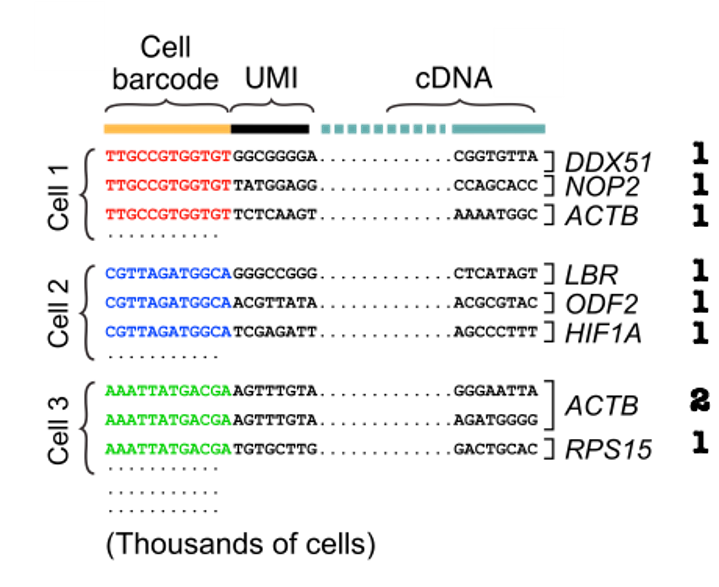
Figure 1.4: Grouping barcodes to assign reads to cells (modified from David Tse et.al)
Analysis of the cell barcodes and UMIs is included in the alignment process, and we will introduce more in Chapter 2.
**Learning Assessment**
1. What is the difference between a cell barcode and UMI barcode? and what are their significance?
2. What are the lengths of the cell barcode and UMI barcode used in our dataset?
3. Are these barcodes located on the 5' or 3' read file?
1.4 Summary of widely used scRNA-seq technologies
Following is a summary table from (Chen, Ning, and Shi 2019). It shows different features of widely used scRNA-seq technologies.
Methods | Transcript coverage | UMI possibility | Strand specific | References |
---|---|---|---|---|
Tang method | Nearly full-length | No | No | Tang et al. (2009) |
Quartz-Seq | Full-length | No | No | Sasagawa et al. (2013) |
SUPeR-seq | Full-length | No | No | X. Fan et al. (2015) |
Smart-seq | Full-length | No | No | Ramsköld et al. (2012) |
Smart-seq2 | Full-length | No | No | Picelli et al. (2013) |
MATQ-seq | Full-length | Yes | Yes | Sheng et al. (2017) |
STRT-seq STRT/C1 | 5′-only | Yes | Yes | Islam et al. (2011) |
CEL-seq | 3′-only | Yes | Yes | Hashimshony et al. (2012) |
CEL-seq2 | 3′-only | Yes | Yes | Hashimshony et al. (2016) |
MARS-seq | 3′-only | Yes | Yes | Jaitin et al. (2014) |
CytoSeq | 3′-only | Yes | Yes | H. C. Fan, Fu, and Fodor (2015) |
Drop-seq | 3′-only | Yes | Yes | Macosko et al. (2015) |
InDrop | 3′-only | Yes | Yes | Klein et al. (2015) |
Chromium | 3′-only | Yes | Yes | Zheng et al. (2017) |
SPLiT-seq | 3′-only | Yes | Yes | Rosenberg et al. (2018) |
sci-RNA-seq | 3′-only | Yes | Yes | Cao et al. (2017) |
Seq-Well | 3′-only | Yes | Yes | Gierahn et al. (2017) |
DroNC-seq | 3′-only | Yes | Yes | Habib et al. (2017) |
Quartz-Seq2 | 3′-only | Yes | Yes | Sasagawa et al. (2018) |
References
Cao, Junyue, Jonathan S Packer, Vijay Ramani, Darren A Cusanovich, Chau Huynh, Riza Daza, Xiaojie Qiu, et al. 2017. “Comprehensive Single-Cell Transcriptional Profiling of a Multicellular Organism.” Science 357 (6352): 661–67.
Chen, Geng, Baitang Ning, and Tieliu Shi. 2019. “Single-Cell Rna-Seq Technologies and Related Computational Data Analysis.” Frontiers in Genetics 10: 317.
Fan, H Christina, Glenn K Fu, and Stephen PA Fodor. 2015. “Combinatorial Labeling of Single Cells for Gene Expression Cytometry.” Science 347 (6222).
Fan, Xiaoying, Xiannian Zhang, Xinglong Wu, Hongshan Guo, Yuqiong Hu, Fuchou Tang, and Yanyi Huang. 2015. “Single-Cell Rna-Seq Transcriptome Analysis of Linear and Circular Rnas in Mouse Preimplantation Embryos.” Genome Biology 16 (1): 1–17.
Gierahn, Todd M, Marc H Wadsworth, Travis K Hughes, Bryan D Bryson, Andrew Butler, Rahul Satija, Sarah Fortune, J Christopher Love, and Alex K Shalek. 2017. “Seq-Well: Portable, Low-Cost Rna Sequencing of Single Cells at High Throughput.” Nature Methods 14 (4): 395–98.
Habib, Naomi, Inbal Avraham-Davidi, Anindita Basu, Tyler Burks, Karthik Shekhar, Matan Hofree, Sourav R Choudhury, et al. 2017. “Massively Parallel Single-Nucleus Rna-Seq with Dronc-Seq.” Nature Methods 14 (10): 955–58.
Hashimshony, Tamar, Naftalie Senderovich, Gal Avital, Agnes Klochendler, Yaron De Leeuw, Leon Anavy, Dave Gennert, et al. 2016. “CEL-Seq2: Sensitive Highly-Multiplexed Single-Cell Rna-Seq.” Genome Biology 17 (1): 1–7.
Hashimshony, Tamar, Florian Wagner, Noa Sher, and Itai Yanai. 2012. “CEL-Seq: Single-Cell Rna-Seq by Multiplexed Linear Amplification.” Cell Reports 2 (3): 666–73.
Hu, Ping, Wenhua Zhang, Hongbo Xin, and Glenn Deng. 2016. “Single Cell Isolation and Analysis.” Frontiers in Cell and Developmental Biology 4: 116.
Hwang, Byungjin, Ji Hyun Lee, and Duhee Bang. 2018. “Single-Cell Rna Sequencing Technologies and Bioinformatics Pipelines.” Experimental & Molecular Medicine 50 (8): 1–14.
Islam, Saiful, Una Kjällquist, Annalena Moliner, Pawel Zajac, Jian-Bing Fan, Peter Lönnerberg, and Sten Linnarsson. 2011. “Characterization of the Single-Cell Transcriptional Landscape by Highly Multiplex Rna-Seq.” Genome Research 21 (7): 1160–7.
Jaitin, Diego Adhemar, Ephraim Kenigsberg, Hadas Keren-Shaul, Naama Elefant, Franziska Paul, Irina Zaretsky, Alexander Mildner, et al. 2014. “Massively Parallel Single-Cell Rna-Seq for Marker-Free Decomposition of Tissues into Cell Types.” Science 343 (6172): 776–79.
Keren-Shaul, Hadas, Ephraim Kenigsberg, Diego Adhemar Jaitin, Eyal David, Franziska Paul, Amos Tanay, and Ido Amit. 2019. “MARS-Seq2. 0: An Experimental and Analytical Pipeline for Indexed Sorting Combined with Single-Cell Rna Sequencing.” Nature Protocols 14 (6): 1841–62.
Klein, Allon M, Linas Mazutis, Ilke Akartuna, Naren Tallapragada, Adrian Veres, Victor Li, Leonid Peshkin, David A Weitz, and Marc W Kirschner. 2015. “Droplet Barcoding for Single-Cell Transcriptomics Applied to Embryonic Stem Cells.” Cell 161 (5): 1187–1201.
Macosko, Evan Z, Anindita Basu, Rahul Satija, James Nemesh, Karthik Shekhar, Melissa Goldman, Itay Tirosh, et al. 2015. “Highly Parallel Genome-Wide Expression Profiling of Individual Cells Using Nanoliter Droplets.” Cell 161 (5): 1202–14.
Picelli, Simone, Åsa K Björklund, Omid R Faridani, Sven Sagasser, Gösta Winberg, and Rickard Sandberg. 2013. “Smart-Seq2 for Sensitive Full-Length Transcriptome Profiling in Single Cells.” Nature Methods 10 (11): 1096–8.
Ramsköld, Daniel, Shujun Luo, Yu-Chieh Wang, Robin Li, Qiaolin Deng, Omid R Faridani, Gregory A Daniels, et al. 2012. “Full-Length mRNA-Seq from Single-Cell Levels of Rna and Individual Circulating Tumor Cells.” Nature Biotechnology 30 (8): 777–82.
Rosenberg, Alexander B, Charles M Roco, Richard A Muscat, Anna Kuchina, Paul Sample, Zizhen Yao, Lucas T Graybuck, et al. 2018. “Single-Cell Profiling of the Developing Mouse Brain and Spinal Cord with Split-Pool Barcoding.” Science 360 (6385): 176–82.
Sasagawa, Yohei, Hiroki Danno, Hitomi Takada, Masashi Ebisawa, Kaori Tanaka, Tetsutaro Hayashi, Akira Kurisaki, and Itoshi Nikaido. 2018. “Quartz-Seq2: A High-Throughput Single-Cell Rna-Sequencing Method That Effectively Uses Limited Sequence Reads.” Genome Biology 19 (1): 1–24.
Sasagawa, Yohei, Itoshi Nikaido, Tetsutaro Hayashi, Hiroki Danno, Kenichiro D Uno, Takeshi Imai, and Hiroki R Ueda. 2013. “Quartz-Seq: A Highly Reproducible and Sensitive Single-Cell Rna Sequencing Method, Reveals Non-Genetic Gene-Expression Heterogeneity.” Genome Biology 14 (4): 1–17.
Sheng, Kuanwei, Wenjian Cao, Yichi Niu, Qing Deng, and Chenghang Zong. 2017. “Effective Detection of Variation in Single-Cell Transcriptomes Using Matq-Seq.” Nature Methods 14 (3): 267–70.
Tang, Fuchou, Catalin Barbacioru, Yangzhou Wang, Ellen Nordman, Clarence Lee, Nanlan Xu, Xiaohui Wang, et al. 2009. “MRNA-Seq Whole-Transcriptome Analysis of a Single Cell.” Nature Methods 6 (5): 377–82.
Tse, David. 2018. “Lecture 16: Single Cell Rna-Seq - Introduction.” Data Science for High-Throughput Sequencing. http://data-science-sequencing.github.io/Win2018/lectures/lecture16/.
Zheng, Grace XY, Jessica M Terry, Phillip Belgrader, Paul Ryvkin, Zachary W Bent, Ryan Wilson, Solongo B Ziraldo, et al. 2017. “Massively Parallel Digital Transcriptional Profiling of Single Cells.” Nature Communications 8 (1): 1–12.
For more information: http://data-science-sequencing.github.io/Win2018/lectures/lecture16/↩︎